views
The Technology Behind Wimbo App's Matching System
Introduction to Wimbo’s Matching Philosophy
In an era where digital connections often feel impersonal and overwhelming, the Wimbo app offers a refreshing alternative by emphasizing meaningful, interest-based networking. Rather than relying solely on superficial parameters like profile pictures or follower counts, Wimbo focuses on mutual compatibility through shared interests, goals, and event participation. The cornerstone of this experience is its intelligent matching system, a blend of algorithms, behavioral data, and real-time analytics designed to forge genuine human connections. This article explores the innovative technology behind Wimbo’s unique matchmaking architecture and how it stands apart in the saturated world of social networking apps.
Interest-Based User Profiling
At the heart of Wimbo's system is a robust user profiling engine that prioritizes user preferences and interests. When users first join the platform, they are prompted to select from a diverse list of hobbies, causes, event types, and lifestyle categories. This data forms the initial layer of each user’s interest profile. Unlike traditional platforms that may use this information only for content suggestions, Wimbo integrates it into its matching algorithm as a fundamental component.
The system doesn’t just store these interests statically. It updates dynamically as users interact with various features in the app—such as browsing events, engaging in discussions, or liking content—thereby refining its understanding of the user's real-time preferences. This dynamic profiling is essential for producing matches that remain relevant over time, even as users’ interests evolve.
AI-Powered Compatibility Scoring
Wimbo's matching system is driven by an AI-powered compatibility scoring mechanism that continuously evaluates potential connections based on multifaceted criteria. At the core of this engine is a machine learning model trained on thousands of anonymized user interaction patterns. These include not only declared interests but also inferred data such as event participation rates, communication style, response time, and shared mutual connections.
The system uses natural language processing (NLP) to analyze user bios, post content, and even chat histories (with user consent) to detect emotional tone, communication frequency, and conversational alignment. These elements are then synthesized into a compatibility score—an abstract numerical value that determines how well two users might connect in a real-world or digital setting. This score drives the order in which profiles are shown and the types of group events users are recommended to join.
Geo-Social Contextual Matching
Beyond shared interests and compatibility scoring, Wimbo adds another layer of intelligence through geo-social contextual matching. This feature considers not only the users' physical proximity but also their past behavior within specific geographic zones. For instance, if two users frequently attend music events in the same neighborhood or have visited overlapping venues, Wimbo’s algorithm will elevate the likelihood of a match.
This is powered by a hybrid geofencing and GPS-based model that detects user activity patterns within defined zones. The app employs lightweight, privacy-compliant location tracking to ensure that suggested connections are not only compatible but also conveniently accessible. By embedding this local context, Wimbo enhances the chances of users meeting up offline, aligning perfectly with its goal of turning virtual connections into real-life interactions.
Real-Time Behavior Analytics
A defining strength of Wimbo’s matching system is its use of real-time behavior analytics. While most social networking apps rely on static profiles, Wimbo constantly evaluates live user behaviors to deliver more precise and timely matches. This includes monitoring when users are most active, what types of content they interact with, and how they navigate different sections of the app.
The platform uses event stream processing technology to analyze these behaviors in real time. For instance, if a user who typically engages with travel-related content suddenly starts exploring technology events, the algorithm will temporarily prioritize matches that align with this new interest. This dynamic responsiveness makes the user experience feel more intuitive and tailored.
Feedback Loops and Algorithm Refinement
Wimbo’s development team places strong emphasis on continuous improvement through algorithmic feedback loops. After every significant user action—such as swiping right, joining an event, or chatting with a match—the system captures engagement metrics and interprets them as signals for refining future recommendations. If a user tends to have longer conversations with matches who share a certain type of interest, the algorithm adapts to prioritize those interests more heavily in future match considerations.
These feedback loops are governed by reinforcement learning models, where the system is “rewarded” when user interactions result in positive outcomes such as mutual swipes, successful event meetups, or ongoing communication. This machine learning method allows the system to self-optimize without requiring manual intervention, ensuring continuous growth in matching accuracy.
Trust and Safety Protocols
With any AI-driven matching system, trust and safety are paramount. Wimbo integrates advanced safety protocols directly into its algorithmic framework to minimize the risk of harassment, misrepresentation, or unwanted attention. The app employs automated moderation tools that screen for inappropriate language or patterns of behavior that deviate from community standards.
Moreover, the app includes a reputation scoring system that factors user behavior into match visibility. Users who receive consistent positive feedback—such as being marked as respectful, responsive, or enjoyable to talk to—are given a higher trust rating. Conversely, users flagged for inappropriate behavior are either demoted in the matching algorithm or suspended based on severity. This trust layer ensures that the algorithm promotes respectful and meaningful interactions.
Event-Driven Group Matching
A unique feature of Wimbo’s matching system is its focus on event-driven group interactions. Unlike one-to-one matching apps that isolate users into private chats, Wimbo encourages community-building through shared event participation. The app’s algorithm intelligently clusters users into groups based on overlapping interests, schedules, and event preferences.
For example, when a local book club event is posted, the system identifies users who have shown interest in literature, reading events, or similar gatherings. It then sends invitations based on both group synergy and past interaction patterns. This approach enables users to connect in more organic, communal settings rather than relying solely on individual conversations.
Integration with Third-Party APIs
To enhance the richness of its matching system, Wimbo integrates with third-party APIs such as event aggregators, calendar apps, and even wellness platforms. These integrations allow users to sync their schedules, import event data, and receive personalized suggestions based on activities outside the Wimbo ecosystem.
For instance, if a user’s Google Calendar shows a preference for fitness classes on Saturday mornings, the Wimbo app might prioritize introducing them to a wellness group that meets around the same time. By bridging data across platforms, Wimbo enhances personalization while maintaining user control over privacy settings.
The Future of Wimbo’s Matching System
Wimbo’s roadmap includes even more sophisticated personalization features, including AI-driven sentiment tracking, conversational intelligence bots, and augmented reality social previews. The development team is also exploring federated learning—a method that allows AI to be trained on-device without uploading personal data to centralized servers. This promises to increase both accuracy and privacy.
Future iterations may also include integration with wearable tech, enabling the app to gauge mood or energy levels to suggest social activities that align with a user’s current state. As Wimbo continues to evolve, its matching system is expected to become increasingly nuanced, empathic, and deeply attuned to the complexities of human interaction.
Conclusion
The Wimbo app stands at the intersection of social innovation and technological precision. Its matching system, built on a foundation of interest profiling, AI compatibility scoring, behavioral analytics, and trust protocols, is more than a technical framework—it’s a dynamic ecosystem designed to foster real human connection. In a digital age where loneliness can persist despite constant connectivity, Wimbo’s thoughtful application of technology offers a meaningful and much-needed alternative. By continually refining its algorithms with user-centric data and ethical AI practices, Wimbo is setting a new benchmark for the future of social networking.
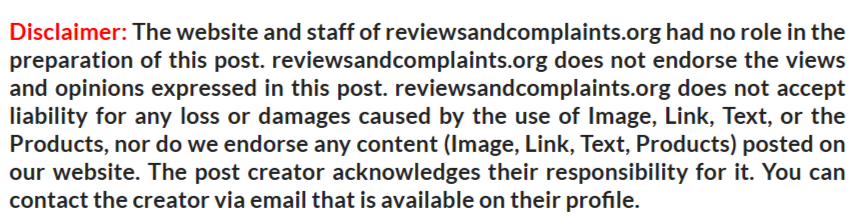
Comments
0 comment